The Importance of Semantic Segmentation Labeling Tools in Software Development
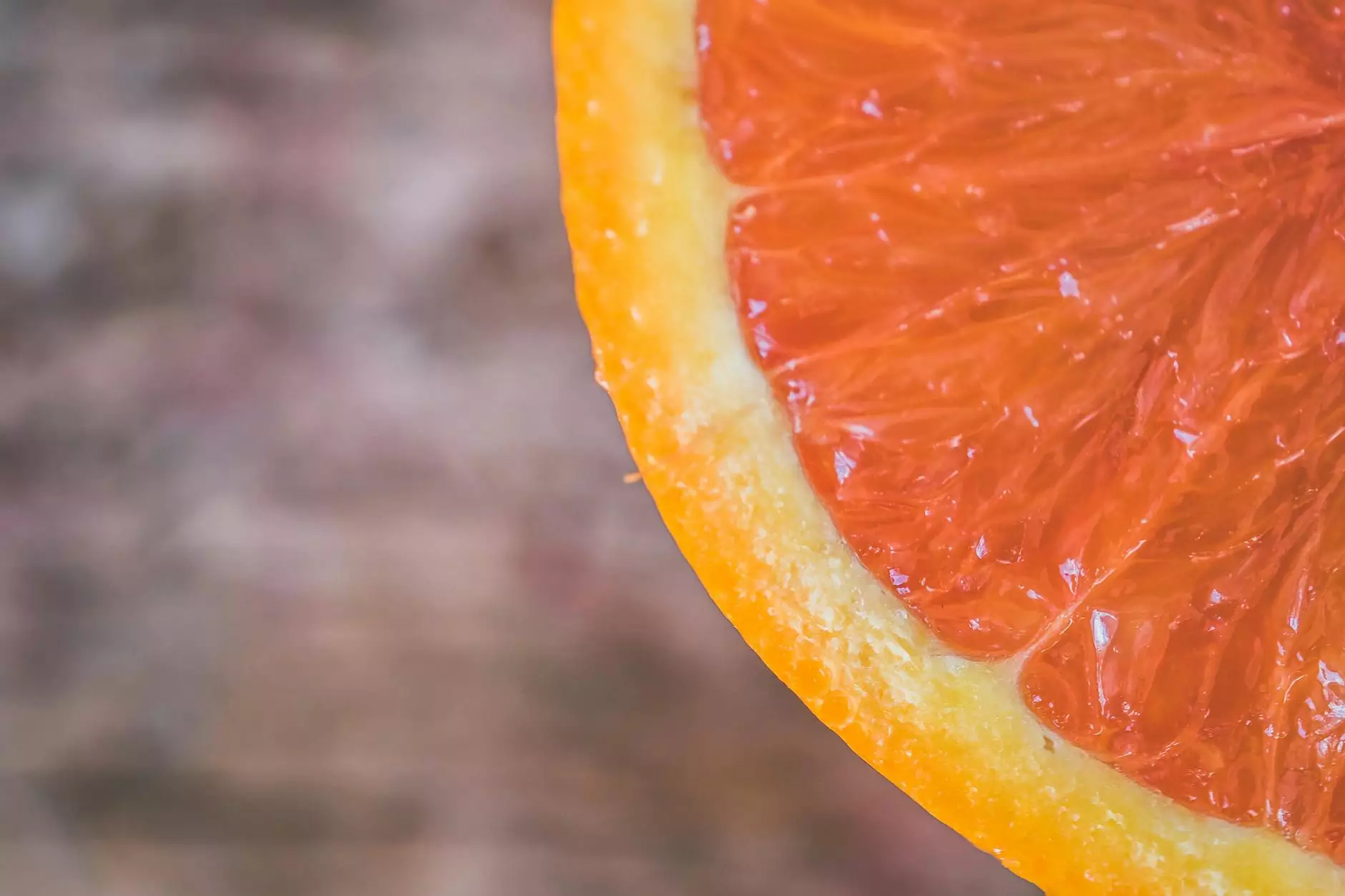
In today's digital landscape, the emergence of semantic segmentation labeling tools has paved the way for innovative advancements in various fields, especially within software development. These tools play a crucial role in the realm of artificial intelligence (AI) and machine learning (ML) by facilitating the training of sophisticated models. This article delves into the significance of semantic segmentation and how the right labeling tools can streamline processes, enhance precision, and ultimately drive business success.
Understanding Semantic Segmentation
Semantic segmentation refers to the process of classifying the pixels in an image into predefined categories or classes. Unlike traditional image classification, which labels an entire image, semantic segmentation provides detailed information about the individual parts of an image. For instance, in a street scene, a model could identify pixels belonging to cars, pedestrians, buildings, and the sky, thus painting a comprehensive picture of the environment.
Key Advantages of Semantic Segmentation
- Enhanced Accuracy: By providing pixel-level classification, semantic segmentation significantly improves the accuracy of computer vision systems.
- Greater Detail: This technique enables AI systems to interpret complex scenes with remarkable fidelity, essential for applications like autonomous driving.
- Improved Data Utility: Semantic segmentation maximizes the utility of image data by transforming raw visuals into structured information.
The Role of Labeling Tools in Semantic Segmentation
To effectively leverage semantic segmentation, businesses need robust labeling tools. A semantic segmentation labeling tool assists data scientists and developers in annotating images with precision and ease. The quality of annotated data directly influences the performance of machine learning models, highlighting the necessity of sophisticated labeling solutions.
Characteristics of Effective Labeling Tools
When considering semantic segmentation labeling tools, several key characteristics distinguish superior solutions:
- User-Friendly Interface: A straightforward, intuitive design allows users of all skill levels to navigate the tool effectively.
- Efficient Annotation Features: Features such as auto-segmentation, quick labeling shortcuts, and undo functionalities can vastly improve productivity.
- Collaboration Support: Tools that facilitate teamwork through real-time collaboration and feedback mechanisms are essential for successful projects.
- Export Capabilities: The ability to seamlessly export data in various formats compatible with different machine learning frameworks is critical.
Choosing the Right Semantic Segmentation Labeling Tool
With numerous options available in the market, selecting the right semantic segmentation labeling tool can be daunting. Here are some factors to consider when making your choice:
1. Project Requirements
Evaluate the specific needs of your project. Consider factors such as the complexity of the images, volume of data, and the granularity of segmentation required. For instance, projects in medical imaging may require more detailed annotations than those in general landscape analysis.
2. Integration with Existing Workflows
Choose a tool that integrates smoothly with your existing data pipelines and software development processes. This pairing prevents bottlenecks and enhances overall efficiency.
3. Scalability
As your project grows, your chosen labeling tool should also scale appropriately. Ensure that it can handle increased volumes of data and more complex labeling requirements as your needs evolve.
Best Practices for Using Semantic Segmentation Labeling Tools
To maximize the value obtained from semantic segmentation labeling tools, follow these best practices:
- Train Your Team: Invest time in training your team to proficiently use the chosen tools, ensuring they grasp all features and functionalities.
- Establish Clear Guidelines: Develop comprehensive guidelines for segmentation tasks to maintain consistency across all annotations.
- Conduct Regular Reviews: Implement periodic reviews of annotated data to ensure quality and make necessary adjustments based on feedback.
- Utilize Feedback Loops: Establish mechanisms for continuous improvement by utilizing feedback from model performance to refine labeling accuracy.
Applications of Semantic Segmentation Labeling Tools
The implementation of semantic segmentation labeling tools extends across various industries and applications. Here are some notable uses:
1. Autonomous Vehicles
In the automotive sector, semantic segmentation is pivotal for enabling autonomous driving systems. Cars equipped with advanced AI algorithms rely on precise visual understanding to detect and react to road conditions, obstacles, and traffic signs.
2. Healthcare
In medical imaging, semantic segmentation assists in identifying tumors, organs, and other critical structures to enhance diagnostic accuracy and treatment planning.
3. Robotics
Robots equipped with vision systems use semantic segmentation to interact intelligently with their environments. This capability allows for tasks such as sorting objects by category or navigating complex spaces.
4. Environmental Monitoring
Semantic segmentation tools assist environmental scientists in classifying land use, assessing deforestation, and monitoring wildlife habitats, thus facilitating better conservation strategies.
Future Trends in Semantic Segmentation
As technology continues to evolve, the field of semantic segmentation is poised for significant advancements. Here are some trends to watch for:
1. Improved Algorithms
The explosion of deep learning techniques has already transformed semantic segmentation. Future developments will likely produce even more sophisticated algorithms that increase accuracy while reducing the need for extensive labeled datasets.
2. Enhanced User Experiences
Emerging tools will continue to focus on enhancing user experience through intuitive designs, customizable features, and advanced automation to alleviate the burden of manual labeling.
3. Integration with Other Technologies
Expect to see more semantic segmentation labeling tools integrate seamlessly with other AI-powered technologies such as augmented reality and virtual reality, broadening their applications in new fields.
Conclusion
In conclusion, the significance of semantic segmentation labeling tools in software development cannot be overstated. As businesses strive to harness the full potential of AI, the accuracy and quality of data labeling will play a decisive role in success. By adopting the right tools, following best practices, and keeping abreast of industry trends, organizations can position themselves at the forefront of innovation in this rapidly evolving landscape.
If you're looking to elevate your software development projects with top-tier semantic segmentation labeling tools, visit keymakr.com to explore cutting-edge solutions tailored for success.