Unlocking the Power of Image Annotation Tools for Machine Learning
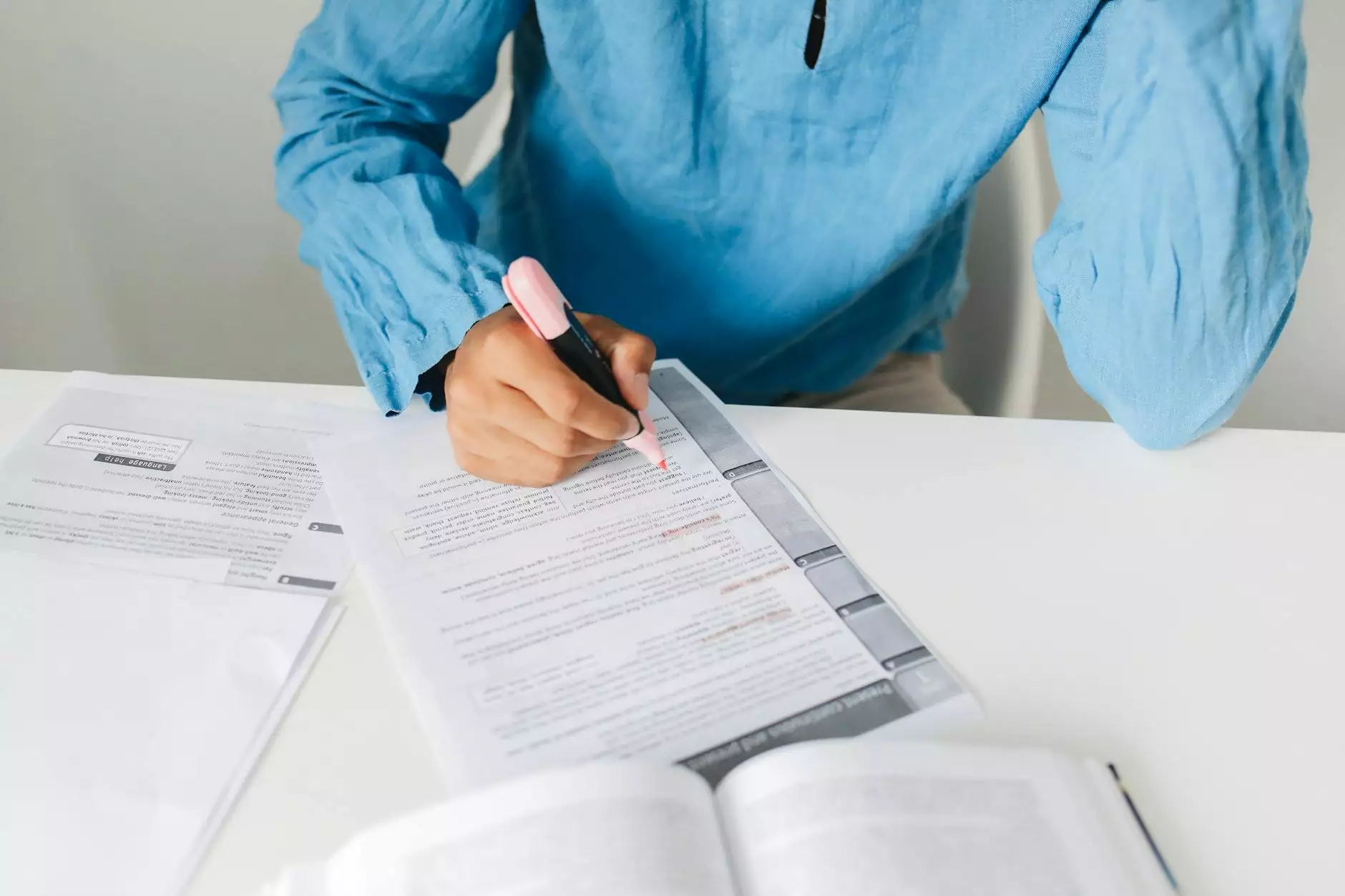
In today's digital landscape, machine learning has emerged as a transformative force across various industries. From healthcare to autonomous vehicles, the need for high-quality data to fuel machine learning models is paramount. One of the most critical aspects of this data is accurate and effective image annotation. This article delves into the significance of image annotation tools for machine learning, exploring how platforms like Keylabs.ai can enhance the annotation process, drive innovation, and ultimately contribute to the success of machine learning projects.
The Importance of Image Annotation in Machine Learning
Machine learning algorithms rely heavily on data input, and the accuracy of these algorithms is directly proportional to the quality of the input data. Image annotation serves as a bridge between raw images and intelligent analysis. Without proper annotation, machine learning models may misinterpret data, leading to flawed predictions and outcomes. Here are some critical reasons why image annotation is vital:
- Enhanced Model Accuracy: Properly annotated images train models to recognize patterns and make accurate predictions.
- Domain-Specific Training: Different domains (like healthcare, gaming, etc.) require specific annotations, ensuring models understand the context.
- Reduction of Bias: High-quality annotation helps mitigate biases in model training by providing a diverse dataset.
- Automation and Efficiency: Advanced annotation tools automate tedious tasks, allowing data scientists to focus on model performance.
Understanding Image Annotation Tools
Image annotation tools are software applications designed to label images for machine learning projects accurately. These tools facilitate creating datasets by enabling users to mark specific features in images, such as objects, boundaries, or even facial expressions. The choice of an annotation tool can significantly influence the efficiency of the data labeling process. Here are key features to consider:
1. User-Friendly Interface
A streamlined, intuitive interface is essential. It enables both novice and experienced users to navigate the tool effortlessly, reducing the learning curve and increasing productivity.
2. Compatibility with Various Data Formats
Advanced annotation tools support multiple file formats (JPEG, PNG, TIFF, etc.), ensuring flexibility in use. The ability to work with different formats helps data teams gather data from multiple sources.
3. Real-Time Collaboration
Collaboration features allow teams to work together in real-time, making it easier to share insights, provide feedback, and ensure consistent annotation quality.
4. Customizable Annotation Types
The best tools offer various annotation types (bounding boxes, polygons, key points) to accommodate the specific needs of different machine learning applications.
5. Quality Assurance Mechanisms
Implementing quality assurance processes ensures that the annotations are accurate and reliable, which is crucial for the success of machine learning initiatives.
Keylabs.ai: Revolutionizing Image Annotation
Keylabs.ai stands out as a leader in delivering intuitive and robust data annotation tools. With a clear focus on quality and efficiency, Keylabs.ai offers a comprehensive platform that enhances the annotation process for machine learning projects. Here’s how Keylabs.ai can transform your image annotation experience:
1. High-Quality Annotations
Quality is paramount in machine learning. Keylabs.ai employs a combination of automated algorithms and expert annotators to ensure that every image is annotated with the highest precision. This dual approach alleviates common errors found in purely automated systems while speeding up the process.
2. Scalability
Whether you're managing a small dataset or training models with millions of images, Keylabs.ai scales with your needs. The platform can accommodate projects of varying sizes, ensuring that your data requirements are met efficiently.
3. Advanced Annotation Techniques
Keylabs.ai provides several annotation methods, including semantic segmentation, instance segmentation, and even custom tool creation for specific project requirements. This versatility ensures your models receive the diverse training necessary for accurate predictions.
4. Robust Integration Capabilities
For businesses that leverage existing tools, Keylabs.ai offers robust integration options with various machine learning and data processing platforms. This capability streamlines the workflow and enhances productivity.
5. Extensive Support and Resources
Keylabs.ai is not just a tool; it’s a comprehensive solution with dedicated support. Through tutorials, documentation, and customer service, users are never left in the dark, ensuring successful implementation of annotation projects.
Best Practices for Image Annotation
To maximize the effectiveness of your image annotation efforts, consider the following best practices:
1. Define Clear Annotation Guidelines
Establish detailed guidelines on how images should be annotated. This includes instructions on labeling, handling edge cases, and ensuring consistency across different annotators.
2. Train Your Annotators
Regardless of whether you’re using automatic or manual annotation, providing training sessions for your team can improve the quality of annotations significantly.
3. Use Iterative Approaches
Implement an iterative annotation process, allowing for ongoing improvements based on feedback and insights derived from model performance.
4. Monitor and Evaluate Annotation Quality
Regularly review annotated datasets to identify trends or recurrent errors, and address any issues proactively to maintain high data quality.
5. Leverage Automation Wisely
While automation can enhance speed, relying solely on automated tools without human oversight can lead to inaccuracies. Enjoy the balance between automation and human intelligence.
The Future of Image Annotation Tools in Machine Learning
As machine learning continues to evolve, so too do the demands placed on data annotation tools. The future of image annotation is poised for exciting developments, including:
- Increased Automation: Advances in AI will continue to supply annotation tools that offer increased levels of automation while ensuring accuracy.
- Real-Time Performance Analysis: Tools will increasingly incorporate real-time performance analytics to provide immediate feedback on the effectiveness of annotations.
- Integration with Augmented Reality: Future tools may harness augmented reality to assist in visualizing data and improving the annotation environment.
- Enhanced Collaborative Technologies: Continued evolution in collaborative tools will further optimize remote work scenarios as teams spread across geographies can easily annotate and provide real-time feedback.
- Focus on Ethical AI: As machine learning continues to encroach into sensitive areas (like surveillance and healthcare), tools will likely incorporate ethical guidelines in the annotation process to prevent bias.
Conclusion
In conclusion, the role of image annotation tools for machine learning cannot be overstated. With platforms like Keylabs.ai, businesses can ensure high-quality data inputs that are pivotal for training successful machine learning models. By understanding the best practices, leveraging the right tools, and keeping abreast of industry advancements, organizations can drive innovation and stay competitive in an ever-evolving technological landscape. Investing in effective image annotation strategies today will undoubtedly pay dividends for machine learning implementations tomorrow.
image annotation tool for machine learning